A single cart of perfectly ripe tomatoes is far more valuable and useful to a chef than a million carts of tomatoes that have gone bad.
Similarly, in the realm of machine learning models, a set of high-quality data fed into the model has the potential to unlock far superior results in generating AI creations compared to a massive quantity of low-quality or outdated data.
Generative AI has emerged as a game changer, quickly making its way into the mainstream. Businesses have harnessed its power to expedite time-consuming tasks, liberating valuable time to seek and seize new avenues for growth; however, we are merely scratching the surface of what this AI application can do.
To dig deeper, organizations need to look at the foundational ingredient for machine learning models – data. With generative AI, the quality of the data fed outweighs the quantity of data available. Just like tomatoes…
How then can businesses curate high quality data, to make better and faster business decisions? At the sidelines of Denodo’s series of DataFest 2023 events, DigiconAsia posed this question – and more – to Angel Viña, CEO and Founder, Denodo.
With AI taking business strategies by storm, and the quality of data being critical to AI, what are the key data management challenges businesses face in an AI-driven era?
Viña: In the landscape of data management, organizations encounter a trifecta of formidable challenges: volume, real-time processing, and data privacy/security.
As AI adoption becomes more prevalent among organizations, this phenomenon will further contribute to the already substantial amount of existing data. This sheer amount of data across multiple systems will pose challenges to businesses, preventing them from leveraging their data efficiently and effectively.
For supervised learning AI models, data labeling and annotation can be time-consuming and costly, especially when working with extensive datasets.
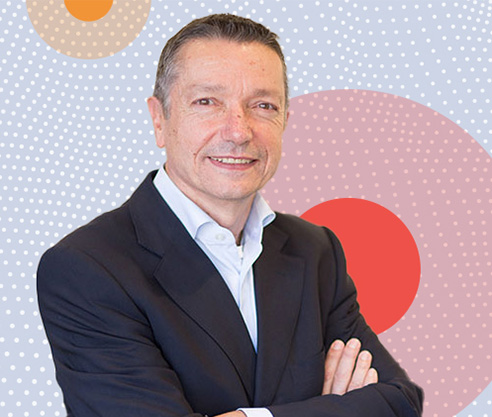
Moreover, AI applications often demand real-time data processing capabilities to provide instant insights and make rapid decisions. Without an efficient data management system, organizations will face difficulties in quickly retrieving accurate and reliable data to feed into their learning models.
Data privacy and security are also critical concerns as AI involves handling sensitive information. Failing to implement robust security measures leaves these datasets vulnerable to breaches and unauthorized access. This poses a significant risk to businesses, as data breaches can lead to severe consequences, including reputational damage and legal ramifications.
What are some ways businesses can navigate the data management concerns brought about by generative AI?
Viña: Amidst the array of challenges, organizations have a plethora of strategic options. Notably, data quality management tools serve as vigilant guardians, detecting and rectifying data imperfections through profiling, cleansing, and deduplication. This ensures that AI applications thrive on a foundation of precise, comprehensive, and dependable data.
Moreover, data integration tools can harmoniously unify diverse data streams, molding them into a coherent format for insightful AI analysis, ultimately empowering organizations to conquer the complexities of the data-driven landscape.
While these are important elements that can help organizations unlock the solution to the problem, ultimately organizations must refine their data management strategy. This helps to ensure that the organization’s approach to manage, leverage, and utilize data can enable it to achieve its business objectives.
The data strategy should place a strong emphasis on data governance, quality, privacy, and security, while keeping close alignment to the organizations’ business goals.
How should businesses transform their data management strategy in the face of such challenges?
Viña: When delving into the essence of a robust data strategy, organizations can initiate by nurturing a data-driven ethos, wherein data becomes an inseparable part of the organizational fabric, shaping every business decision.
This entails establishing comprehensive end-to-end data accessibility across the entire company, dismantling data silos, and ensuring swift availability of vital data to those who require it and when they require it. This would not only foster well-informed decisions but also mitigates the peril of relying on outdated or incomplete information.
To facilitate this, organizations should consider implementing self-service analytics tools and platforms. These intuitive instruments empower employees to autonomously access and analyze data, without relying solely on data specialists or IT teams. By providing training and support to employees on how to effectively use these tools, organizations can instill a sense of data empowerment, where individuals feel confident in handling and interpreting data themselves.
This approach not only streamlines decision-making processes but also encourages a culture of curiosity and exploration. Employees become more engaged with data, uncovering valuable insights and innovative solutions to challenges. Data-driven decision-making becomes a natural part of the organizational fabric, guiding strategies, operations, and actions across all levels.
How can organizations leverage data virtualization tools to curate a set of high-quality data?
Viña: High-quality data exemplifies the pinnacle of excellence, adhering to the highest standards of accuracy, completeness, reliability, and relevance. Exempt from errors, inconsistencies, and bias, it emerges as a steadfast and invaluable asset for analysis and decision-making, assuring unwavering dependability and unfolding a realm of possibilities.
With data virtualization, business users have access to a holistic view of information, across all the source systems. There is a singular data-access layer established, eliminating the need for physical data movement, while seamlessly handling diverse data formats. This guarantees data accuracy, completeness, and standardization – fostering a reliable foundation for data-driven decisions and analysis.
This centralized and logical layer also enables real-time access to data, which provides organizations with the ability to work with the most up-to-date data that is crucial for time-sensitive applications and decision-making processes.
What is your vision for Denodo Technologies in APAC?
Viña: While most of our revenue today comes from our businesses in North America and Europe, APAC is our fastest-growing region, as we scale our business across ASEAN, Korea, India, ANZ and Greater China Region.
To say the least, I expect much of our growth for the next three to five years to come from the APAC region, as the overall serviceable data management market for us in APAC is huge and most of that is currently untapped.
We are forming very strong partnership with many of the global and regional system integrators, so that together we can solve many of the data management problems for large enterprises, as well as small to medium sized businesses. This vision and growth are also supported by our go-to-market strategy with many technology partners, the latest one being with Alibaba Cloud.
Additionally, we are establishing many local offices all across APAC and hiring sales, services and marketing to propel our growth across the entire region.